Don't have time now? Download it.
Why customers choose Klarity
98% of global executives agree that foundational AI models will play an important role in their organizational strategies over the next 3-5 years, according to Accenture. You’ve likely heard of foundation AI models in the past few months, but under different names: ChatGPT or Google Bard.
They are all Large Language Models (LLMs) – a powerful type of generative AI. We’ve talked a lot about LLMs and how they can help accountants do their jobs. LLMs and generative AI are all the buzz right now, but much of the media coverage focuses on the potential for this technology to replace people rather than to enable them and enhance their working lives.
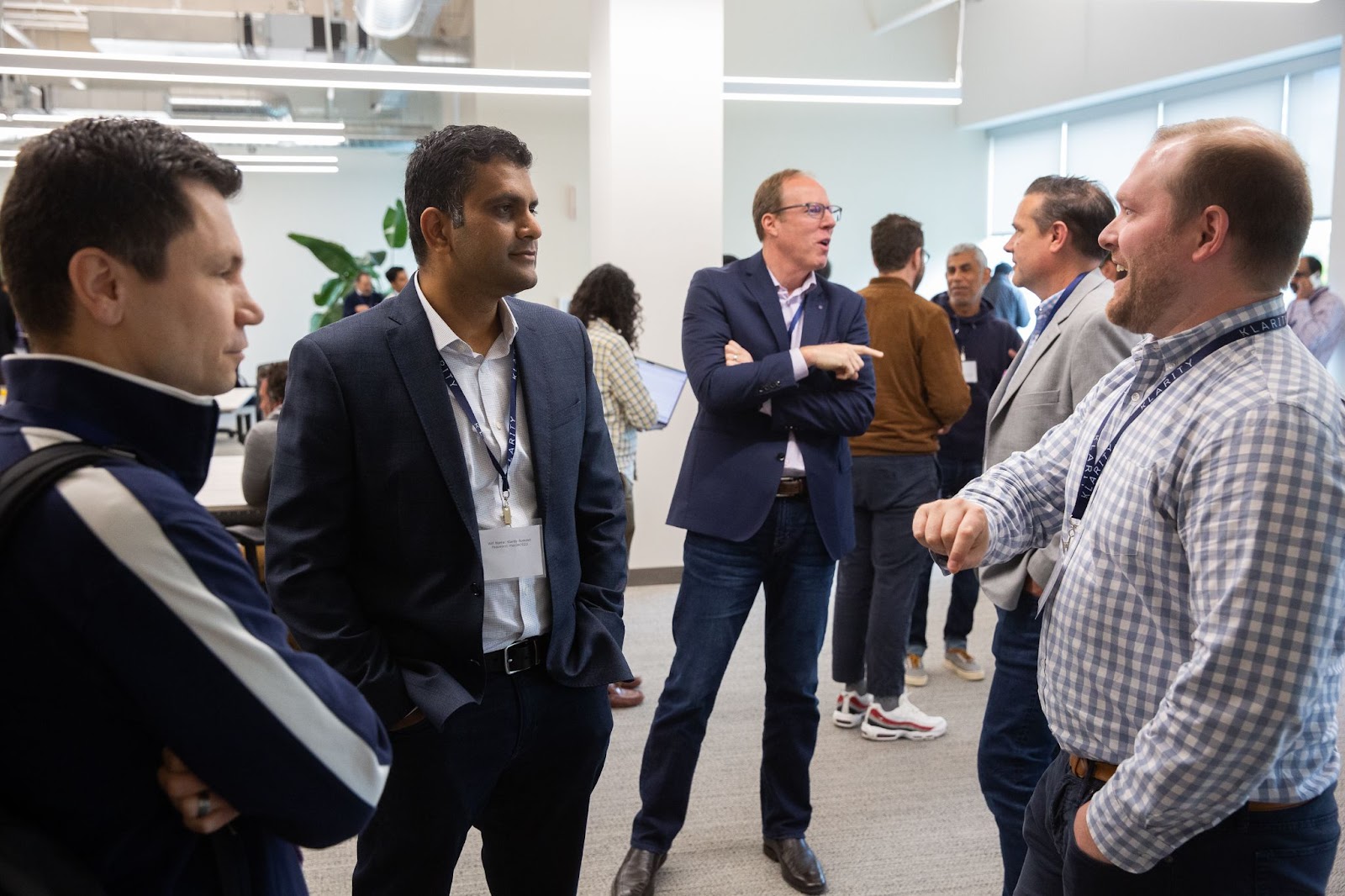
At Klarity, we are as intimately familiar with the limitations of LLMs as we are with their strengths. If you are an accounting leader evaluating LLMs as a solution for workflow automation, there are three common limitations of LLMs to be aware of before you adopt this emerging technology into your revenue operations:
- Hallucinations and Reliability
- Prompt Sensitivity
- Context Window Limits
These issues represent gaps in contextual knowledge and strategic ability that only humans can fill. We see this technology not as a replacement for the accounting pros we work with, but as their best new team member. And as with any other team member, you have to know where their strengths and weaknesses lie.
What are they? How do they manifest, and why? Read on to learn more about these limitations and how they will impact the way B2B accounting professionals work in the next 3-5 years.
Hallucinations and Reliability
“Hallucinations” occur when an AI model fabricates a confident but inaccurate response. This issue can be caused by a number of factors, including divergences in the source content when the data set is incredibly vast, or flaws with how the model is trained. The latter can even cause a model to reinforce an inaccurate conclusion with its own previous responses. It’s not hard to see why that might be a problem for finance and accounting teams. Your work involves mission-critical workflows that demand certainty and repeatability, and a hallucinating AI model represents unacceptable risk when it comes time to recognize revenue on time or reconcile POs with factual data.
Our prior work in document structuring puts up a guardrail against this problem. At Klarity, we built our LLMs with B2B accounting professionals as our primary focus and battle-tested their performance. We’ve developed techniques to ensure that our LLMs perform accurately and reliably, including a variety of prompt design techniques as well as other computational methods such as the use of embedding layers to focus and guide responses. Much of Klarity's pre-existing work in document structuring has helped as well – our ability to represent the text of a document in the way that is most understandable to an LLM makes hallucinations a far less likely occurrence.
Prompt Sensitivity
When working with LLMs there are also significant limitations surrounding prompt engineering, which in its current form can be challenging and inefficient. A prompt is the user input to a Gen AI model, based on which it creates its output. LLMs are highly sensitive to the way prompts are framed. The same idea phrased in three different forms could generate three vastly different responses. OpenAI is actively working to mitigate this issue, and GPT4 suffers far less than its predecessors. However, it is still not entirely resilient to the problem. It’s for this very reason that the role “Prompt Engineer” has been popping up on many company’s hiring pages!
At Klarity we’ve used a number of cutting edge techniques to ensure that our customers can reliably receive high accuracy results. These include adding examples within the prompt so that the Large Language Model can learn from those examples rather than simply relying on the text. We’ve developed sophisticated automated prompt generation pipelines to ensure that prompts are phrased in a statistically optimal way. We’ve also deployed internal tooling to test different prompting regimes at an incredibly high scale and measure accuracy on thousands of data points.
Context Window
The last limitation involves context window size. Expanding the input parameters associated with context windows in LLMs is a significant technical hurdle to overcome. As the amount of text to be considered goes up, as does the computational complexity of the task. GPT-4 has expanded its context window to an astonishing 32,000 tokens– far ahead of the competition– but this limit still puts constraints on the larger, more complex tasks common to document review and accounting workflows. Even the most advanced models can only ingest and analyze a finite amount of information while considering an answer. And a 250-page MSA is beyond the scope of even the most powerful LLMs!
Klarity's Document Chat Feature
But Klarity has cleverly circumvented this obstacle through the creative use of embedding layers. Embeddings are a way of representing content (in this case text) as a simple sequence of numbers, which makes it much quicker to perform other operations. Rather than simply feeding heaps of text into an LLM, Klarity uses an embedding layer to select the portions of a document that are most relevant to a certain query and then only process those. Klarity’s new Document Chat feature is an example of how LLMs have evolved this capability. Teams can now infuse the power of AI models into their individual documents to get their questions answered without moving them off their systems.
Another excellent example of this is Klarity’s new Semantic Search feature. This allows our users to have accurate search functionality, whether it’s identifying non-standard termination for convenience within their documents or confirming the correct billing address within a Purchase Order. It scans every document for contextual meaning related to your search, rather than just keywords alone. And with one click, you can download the results as a CSV. It is a system designed to be easily used and understood by accounting pros, to speed through document and contract review with ease.
Klarity's Semantic Search Feature
What does this all mean for you?
The growth and adoption of LLMs creates a new reality accounting professionals must contend with. There is potential for AI to be inherently good, while its influences do need to be explored with consideration to reap the rewards of it without stumbling over its potential drawbacks. The potential benefits to employing LLMs are such that it will be hard for anyone to opt out of using them entirely, so knowing their limitations will be as critical as understanding where they can help. GenAI will not replace human accountants, but accountants using AI in their daily work will accomplish vastly more and enjoy a better quality of life.To make the latter possible, evaluate areas where you want to use AI to automate lower-level manual efforts in your workflows. Use that time you earn back from AI to enable the higher-level skills unique to financial accountants that will always be critical to do the job.
Life at Klarity
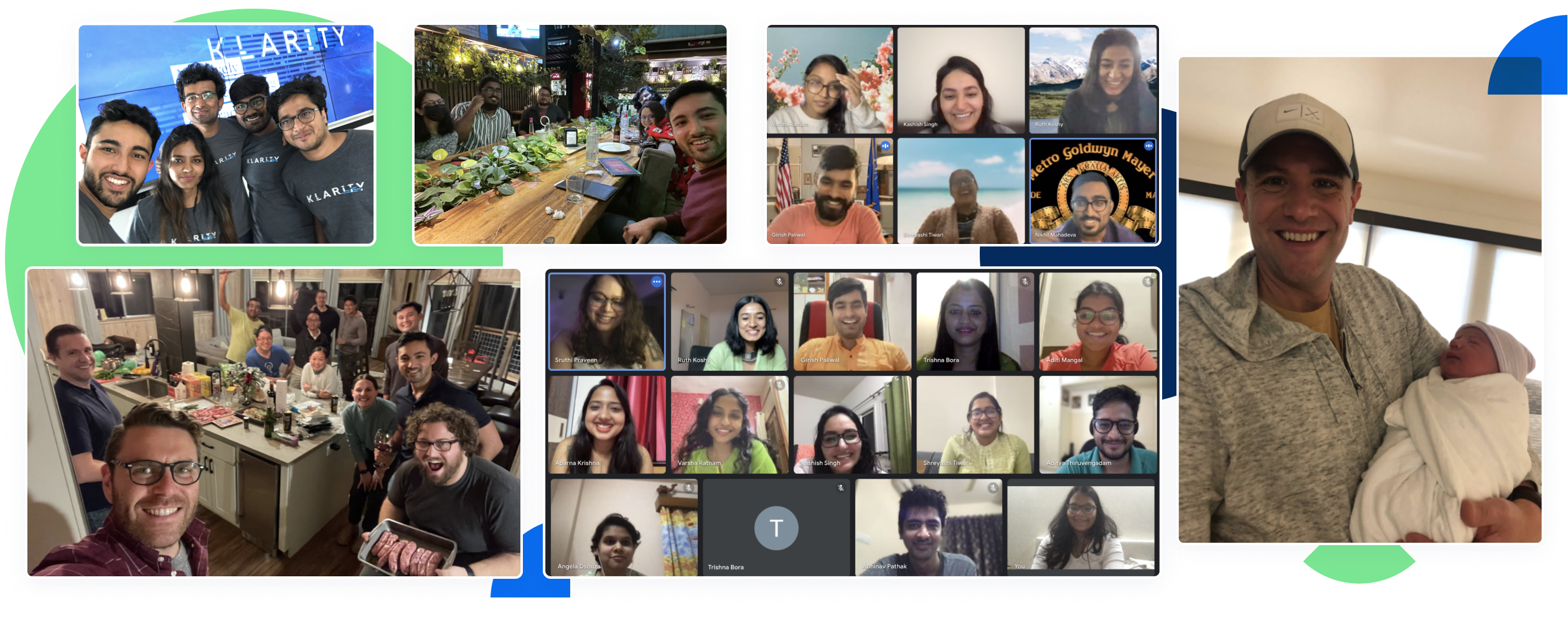
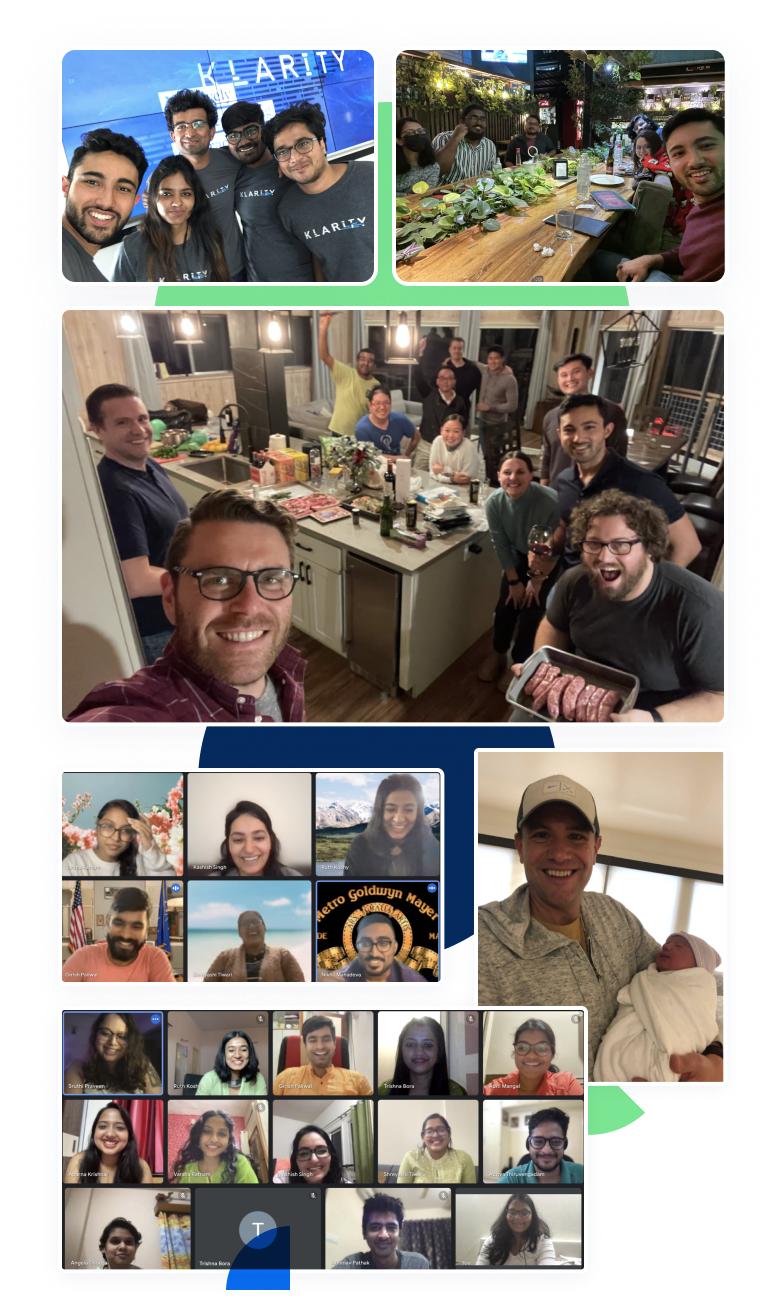